Best Flux Models Comparison Test – Image Quality vs Speed using Forge UI
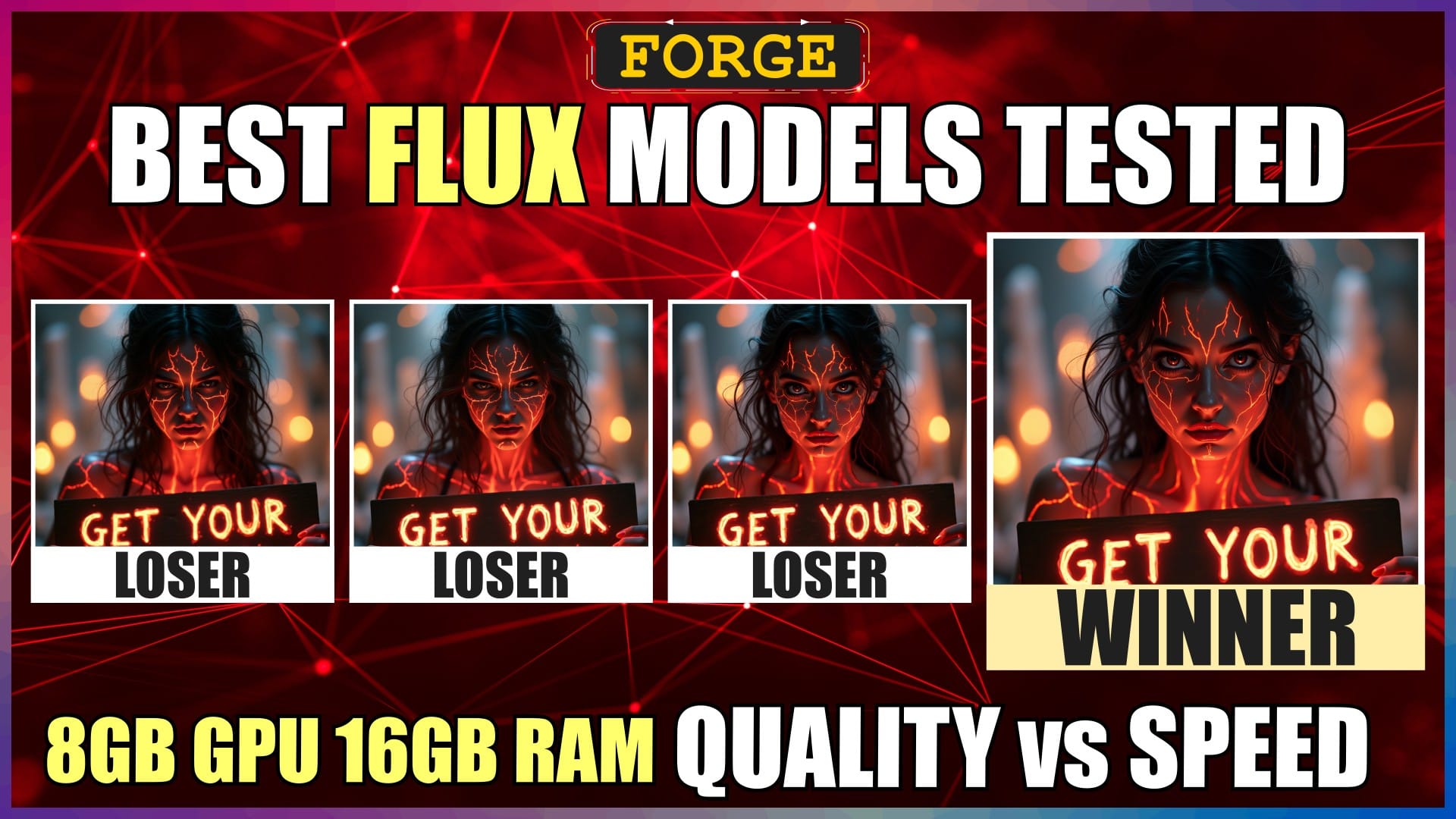
Introduction
Hello there and welcome!
If you’ve wondering about the ACTUAL, like-for-like image quality differences, and generation times, of the most popular Flux models, then this video is for you.
In this video, I’ve selected the 8 most popular Flux models, 4 Schnell models and 4 Dev models, generated a whole bunch of the same images using each of them, so we can compare the results of Schnell vs Schnell, Dev vs Dev and then compare a selection of Schnell and Dev images against each other.
I’ve generated all of these images using Forge UI, with a Windows PC, running an NVIDIA RTX 3070 8GB VRAM GPU, 16GB of RAM and an SSD. This means that all the model generations used my GPU, RAM and SSD virtual memory. So if you’ve got higher or lower spec hardware, you can adjust the generation times up or down accordingly.
This video, is a follow up to my previous Flux video, on how to choose the best Flux model for your own specific needs and hardware, so if you haven’t watched that video yet, then I highly recommend it, it’s got some great tips and insights in it. I’ll leave the link to that video in the description below for you.
So, let’s crack on.
Flux Models Comparison Test Factors
The Schnell and Dev models that I tested for comparison are the FP8, GUFF Q8, NF4 and the GUFF Q4.
For all the models, I used the standard VAE, CLIP and T5 FP8 text encoders.
I’ll leave the links for all of these, in the description below for you.
For the Schnell models, I used 4 sampling steps, and for the Dev models I used 20 sampling steps. It could be argued, that it’s not a fair comparison between Schnell and Dev, using different sampling steps, but I wanted to keep them at their recommended lower end defaults. Schnell would therefore, benefit from faster total generation times, and Dev from perhaps better image quality. Also, increasing Schnell steps to Dev levels, would produce worse image quality results, and lowering Dev steps too far, would generate incomplete images. So 4 and 20 it is.
We’ll start off comparing the Schnell model images to one another, then the Dev model images to one another, then rap up with a quick comparison, of some Schnell and Dev images together.
For the first two detailed comparisons, I’ll be awarding scores out of 5, for overall image quality, overall image prompt adherence and also image text adherence. Bear in mind that my scores are subjective, and based on my opinion, which may obviously differ from yours.
It’s also worth pointing out, that due to a limited sample size, this is by no means a conclusive test. However, it should certainly give you a good indicative comparison.
If you’re only interested in a specific comparison section of the video, then I’ve left the timestamps for each section, in the description below for you, so you can just jump straight to the section you’re interested in.
Flux Schnell Models Comparison
This is the first Schnell model image comparison.
You can see the model generation key details above each image, and the same prompt that was used for all the images at the bottom. I used 4 sampling steps for all the Schnell images.
The Q8 image, took significantly longer to generate than the FP8, and a lot longer than the NF4 and Q4.
The images all seem pretty similar at first glance.
For overall image quality, I’ll give the Q8 image the highest score, of 5 out of 5. The FP8 and Q4 images are pretty much equally good, so I’ll give both a 4. The NF4 image is visibly the lowest in terms of quality, and I’m not sure what’s going on, with the half ring on her finger, so I’ll give it a 3 out of 5.
For overall prompt adherence, I think they all pretty much produced exactly what I asked for, so I’ll give them all, a 5 out of 5.
Text adherence. None of the images got the lower and upper case letters correct. Although the NF4 and Q4 images did get the lower case “t” correct on the “get”, so I’ll give the NF4 and Q4 both a 4, and the FP8 and Q8 both a 3 out of 5.
Moving onto the second image.
This has a longer, more detailed prompt.
For image quality, none of them produced equal or decent fingers. For image detail, the Q8 certainly stands out just ahead of the FP8, so I’ll give the Q8 a 5 and the FP8 a 4. The Q4 has slightly more detail than the NF4, so I’ll give the Q4 a 3 and the NF4 a 2.
Overall prompt adherence, again is pretty good for all the images, so again, I’ll give them all a 5.
For text adherence, they all got the upper and lower case lettering correct this time, but there’s a bit of difference between them. For the FP8 and Q8, I’m not sure where the line in the sign came from, some of the letters extend off the sign, and the FP8 has misspelt the word “hungry”. The Q4 has doubled up on the word “am”. The NF4 has done the best job, so I’ll give it a 5. I’ll give a 4 to the Q8, and a 2 to both the FP8 and Q4.
Moving onto image number 3.
This is a cartoon style image, with a longer text for the sign.
For image quality, there’s quite a bit of funky things happening with all the hands, but apart from that, they’re all pretty good, but the FP8 and NF4 seem better, so I’ll give both of them a 5, and both the Q8 and Q4, a 4 each.
Overall prompt adherence, again, all good, so they all get a 5.
Text adherence, I think is a fail for them all, so only a 2 each.
Onto image 4.
They all did pretty well, except all the fingers are a bit strange, and NF4 is missing one.
So, for overall image quality and detail, I’ll give Q8 a 4, FP8 a 3, and a 2 for both the NF4 and Q4.
For prompt adherence, only the NF4 got the “glimmering crystals in the background”, so I’ll give it a 5, with all the others a 4.
Text adherence, FP8 and Q8 are spot on, so I’ll give them a 5. NF4 is a bit covered, and the Q4 got the wrong letter case for the first letter, so I’ll give them both a 4.
Onto the fifth and final image.
I’m not really impressed with any of them, they all look a bit plastic.
For quality, different images have better details in different parts, so I’m going to go with a 3 for them all.
For overall prompt adherence, again, they all got it, so a 5 for them all.
For text adherence, there’s a wide range of signs and text. Only the Q4 has a misspelling, but none of them got the upper and lower cases correct. FP8 and Q8 get a 4. The NF4 sign design is pathetic, so only a 3, and a 3 for Q4 as well.
OK, that all 5 images compared for each of the 4 Schnell models.
This is a breakdown of the total image generation times and awarded image scores.
The image generation times are probably what you’d expect, with FP8 and Q8, taking a lot longer than NF4 and Q4.
For overall image quality, Q8 and FP8 stand out above NF4 and Q4.
Prompt adherence, they’re all pretty much on an excellent same level.
Text adherence, sees a bit of a lower than expected score for the FP8 model, and a higher than expected score for NF4.
This is a summary of the total image generation times, and total image scores awarded.
Q8 is the overall winner for images, but has considerably longer generation times than any other model.
FP8 is second for images, but a lot quicker for generation times than Q8.
NF4 is surprisingly close to FP8 for images, with the very quickest generation times.
Q4 is last for images, and also slower than NF4 for generation times.
OK, now you know how the different Schnell models stack up against each other.
Flux Dev Models Comparison
This is the first Dev model image comparison.
Again, you can see the model generation key details above each image, and the same prompt that was used for all the images at the bottom. I used 20 sampling steps for all the Dev images.
The images all seem pretty similar at first glance.
For overall image quality, the FP8 and Q8 images have come through with more detail, so I’ll give them both a 5. The Q4 is pretty close with a 4, and the NF4 is just lacking compared to the others, so it only gets a 3.
For overall prompt adherence, they’re all pretty much spot on, so a 5 across the board.
For text adherence, there’s a noticeable adherence to upper and lower case letters compared to the Schnell models, although all the Dev models added a capital “L” in Flux, instead of a lower case “l”. So, a 4 for all images.
Moving on to image number 2, with a longer, more complex prompt.
Image quality. The FP8 and Q8 images seem to have more detail. The fingers and toes have slight issues in all the images. NF4 and Q4 have no eyes. NF4 is noticeably behind the other 3. I’ll give a 4 to FP8 and Q8, a 3 to Q4 and only a 2 to NF4.
Prompt adherence, again spot on for all, so again a 5 for all images.
Text adherence. All upper and lower case correct, and all words correct for all images, so a score of 5 for all images.
Moving on to image number 3.
This is with the longer sign text.
For image quality, they’re all slightly different cartoon styles, which is fine. There’s no obvious issues with any of them, and all are detailed in different areas of the character, so a score of 5 for all images.
For prompt adherence, again, all spot on, so again, a 5 for all.
For text adherence, none are spot on, but the FP8, Q8 and Q4 come pretty close, with the NF4 trailing behind. So, a 3 for the NF4, and a 4 for the rest.
Moving on to image number 4.
For image quality, all the images struggled with the fingers in one way or another. The overall quality of the other details with FP8 and Q8 is equally as good, so a score of 4 for both of them. NF4 and Q4 are visibly lower in quality detail, so a 3 for each of them.
For prompt adherence, I can’t see any crystals in the background, but apart from this, all images adhered to the prompt very well, so a 4 for them all.
For text adherence, none of them got the upper and lower case correct, and they all have the text obscured, so only a score of 3 for all of them.
Moving on to the final image number 5.
Immediately, it’s obvious how much higher quality and more detailed, all of these images are, compared to the Schnell images using the same prompt.
For image quality, the FP8 and Q8 images stand out as better quality with more detail, so a 5 for them both. Between the NF4 and Q4 images, there’s a variety of better details with each, so an even score of 4 for both of them.
For overall prompt adherence, again a 5 across the board.
For text adherence, none of them have the upper and lower case letters correct, but the NF4 sign comes the closest for a score of 4. Next closest is the Q4, for a score of 3. Followed by a score of 2 for both the FP8 and Q8.
OK, that all 5 images compared for each of the 4 Dev models.
This is a breakdown of the total image generation times and awarded image scores.
The difference in image generation times between the models, are pretty much in line with what we saw with the Schnell models, with FP8 and Q8, understandably taking a lot longer than NF4 and Q4.
For overall image quality, Q8 and FP8 jump above NF4 and Q4.
Prompt adherence, they’re all pretty much on an excellent same level.
Text adherence, is surprisingly even between all 4 Dev models, with NF4 and Q4 edging out slightly over FP8 and Q8.
This is a summary of the total image generation times and total image scores awarded.
FP8 and Q8 are tied as the overall winners for images, but Q8 especially, has much longer generation times, than any other model.
Q4 beats NF4 for images, but is slower than NF4 for generation times.
OK, now you know how the different Dev models stack up against each other.
All Flux Schnell and Dev Models Comparison
This is the first image comparing all Schnell and Dev models.
The image dimensions are all 1024 by 1024, and use the exact same prompts. The Schnell models used 4 steps and the Dev models used 20. The spread of image generation times is also pretty similar to the previous comparison numbers.
With this first image set, it’s immediately obvious that the Dev models produce vastly better quality images than the Schnell models.
The quality of the sign text is also much better with the Dev models.
With this second image set, it’s pretty much the same story as the first.
The Dev models produce much better quality and details than the Schnell models.
This is the third image set.
The prompt for this didn’t actually state a cartoon style.
Again, I think the Dev models just outperform the Schnell models overall in terms of quality and detail.
This is the forth and final image set.
You can see even more so with these images, how far ahead the images are with the Dev models compared to Schnell.
The Dev models just contain so much more appropriate detail, and just look a better picture overall.
OK, now you know how all Schnell and Dev models compare to each other.
Conclusion
Now you should have a better insight, into how the most popular Schnell and Dev models stack up against each other, in terms of generation times and a variety of image quality factors.
Anyway, hope you found this video helpful, and I’ll catch you in the next one.
Links
Dev FP16
Model-Only, File = flux1-dev.safetensors
https://huggingface.co/black-forest-labs/FLUX.1-dev/tree/main
Dev FP8
Model-Only, File = flux1-dev-fp8-e4m3fn.safetensors
https://huggingface.co/Kijai/flux-fp8/tree/main
Dev FP8
AIO, File = flux1-dev-fp8.safetensors
https://huggingface.co/Comfy-Org/flux1-dev/tree/main
Dev GUFF Q8
Model-Only, File = flux1-dev-Q8_0.gguf
https://huggingface.co/city96/FLUX.1-dev-gguf/tree/main
Dev GUFF Q4
Model-Only, File = flux1-dev-Q4_0.gguf
https://huggingface.co/city96/FLUX.1-dev-gguf/tree/main
Dev NF4 v2
AIO, File = flux1-dev-bnb-nf4-v2.safetensors
https://huggingface.co/lllyasviel/flux1-dev-bnb-nf4/tree/main
Schnell FP16
Model-Only, File = flux1-schnell.safetensors
https://huggingface.co/black-forest-labs/FLUX.1-schnell/tree/main
Schnell FP8
Model-Only, File = flux1-schnell-fp8-e4m3fn.safetensors
https://huggingface.co/Kijai/flux-fp8/tree/main
Schnell FP8
AIO, File = flux1-schnell-fp8.safetensors
https://huggingface.co/Comfy-Org/flux1-schnell/tree/main
Schnell GUFF Q8
Model-Only, File = flux1-schnell-Q8_0.gguf
https://huggingface.co/city96/FLUX.1-schnell-gguf/tree/main
Schnell GUFF Q4
Model-Only, File = flux1-schnell-Q4_0.gguf
https://huggingface.co/city96/FLUX.1-schnell-gguf/tree/main
Schnell NF4
AIO, File = flux1-schnell-bnb-nf4.safetensors
https://huggingface.co/silveroxides/flux1-nf4-weights/tree/main
VAE
File = ae.safetensors
https://huggingface.co/black-forest-labs/FLUX.1-dev/tree/main
CLIP Text Encoder
File clip_l.safetensors
https://huggingface.co/comfyanonymous/flux_text_encoders/tree/main
T5 Text Encoder
FP8, File = t5xxl_fp8_e4m3fn.safetensors
https://huggingface.co/comfyanonymous/flux_text_encoders/tree/main
T5 Text Encoder
FP16, File = t5xxl_fp16.safetensors
https://huggingface.co/comfyanonymous/flux_text_encoders/tree/main
Related Videos
How to Pick the Best Flux Models for You and Use in Forge UI:
https://youtu.be/DwLdKmnVvoI